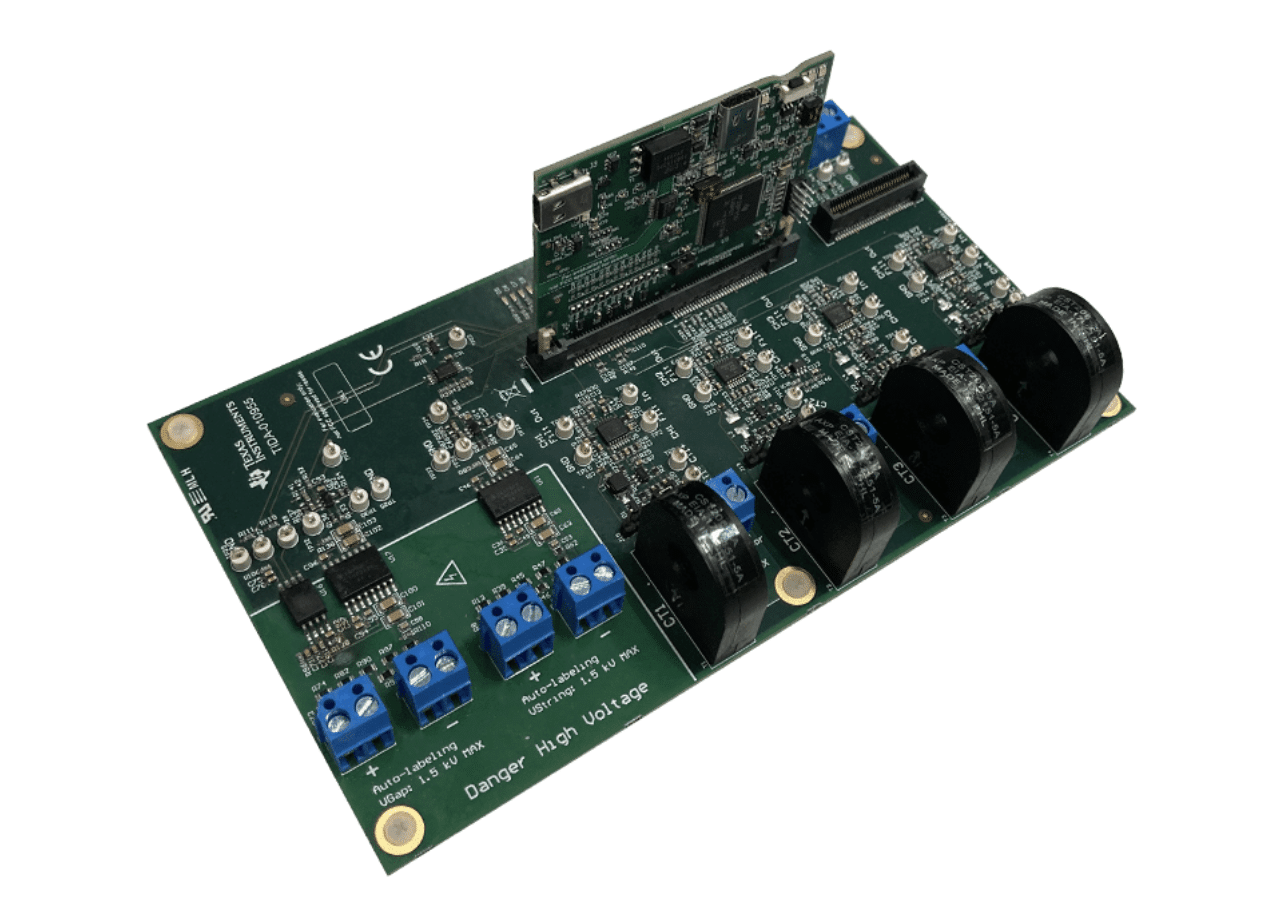
Check out our latest products
The analog front end with AI-based arc detection improves solar safety by detecting arcs and reducing false alarms in photovoltaic systems.
TIDA-010955, a reference design from Texas Instruments (TI) features an analog front end (AFE) for DC arc detection in solar applications, leveraging artificial intelligence (AI) for enhanced performance. The string current is measured, filtered, and processed by an AI-based arc detection algorithm running on the TMDSCNCD28P55X controlCARD, built around the C2000 F28P55x family of devices. The AI approach offers greater accuracy and robustness compared to traditional detection methods. A complete toolchain is provided for collecting arc data, training the embedded AI model, and validating the system. This reference design can be ordered under part number TIEVM-ARC-AFE. The solution is suitable for applications such as solar arc protection, string inverters, and central inverters.
As the number of solar installations continues to rise, ensuring system safety becomes increasingly critical. In particular, arcing on high-voltage lines must be promptly detected, and affected solar strings must be de-energized to prevent hazards such as electrical shock or fire. To address this, standards like UL 1699B require arc-fault protection circuits for all solar systems operating at voltages below 1500V. This reference design presents an implementation of an analog front end specifically for arc detection. However, the design alone does not meet the full requirements of the UL 1699B standard.

DC arcing generates an AC noise current within the photovoltaic (PV) string cabling, spanning a broad frequency range up to several megahertz. The primary challenge of DC arc detection lies in reliably identifying this noise increase without triggering false alarms or unnecessary shutdowns. This demands a low-noise, high-performance analog front end, capable of distinguishing small injected AC noise currents—typically only a few milliamps—superimposed on the much larger DC string current, which can reach around 20A in residential systems and even higher in commercial applications.
Moreover, solar systems are exposed to various other noise sources that must not be mistaken for arcing events, such as inverter switching frequencies and power line communication signals along the PV cabling. Traditionally, detection algorithms have been employed to recognize arc signatures in the current measurements. These algorithms often require system-specific tuning, as arcing signatures tend to vary significantly between installations.
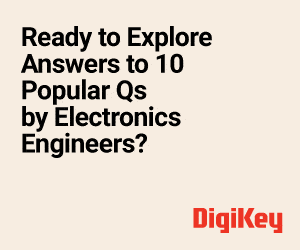
A more recent and promising method involves using embedded Artificial Intelligence (AI) models for arc detection. Properly trained, AI-based solutions can deliver higher detection accuracy and improved resilience against false alarms.
To train these AI models, high-quality labeled data is necessary. For this reason, this reference design incorporates an arc-labeling circuit. It leverages the measurable voltage drop across an arc to distinguish between arcing and normal operating conditions—a method made feasible within controlled laboratory environments where the arc voltage is directly accessible.
TI has tested this reference design. It comes with a bill of materials (BOM), schematics, assembly drawing, printed circuit board (PCB) layout, and more. The company’s website has additional data about the reference design. To read more about this reference design, click here.