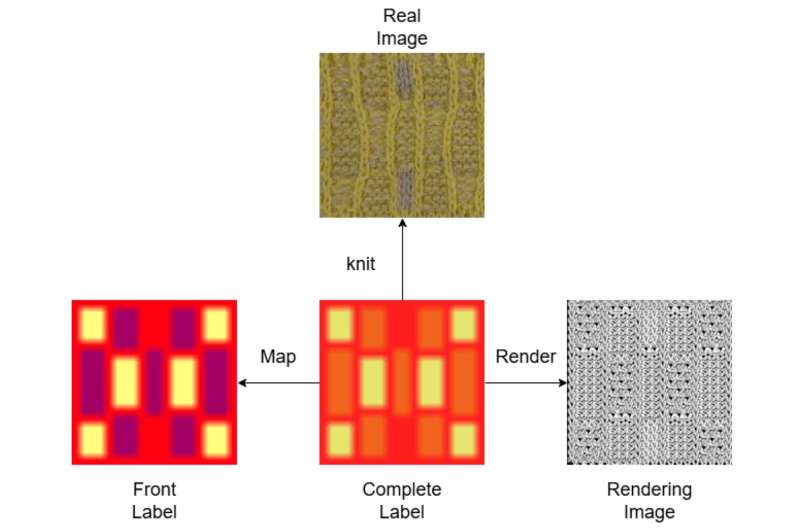
Check out our latest products
The AI system turns fabric pictures into instructions for robots to knit clothes, helping make textile production faster, easier, and more customizable.

Today, many real-world tasks—like those in factories—can now be done by machines, including some parts of making clothes. Researchers at Laurentian University in Canada wanted to see if machines could knit clothes all by themselves. They created a computer program that looks at pictures of fabric and turns them into step-by-step instructions that knitting robots can follow. Their program worked well and was able to help robots knit clothes using one or more yarns.
The newly introduced fabric pattern creation model offers several notable features and advantages. It is capable of generating both single- and multi-yarn knitting patterns, accurately handling rare stitches, and adapting readily to new fabric styles.
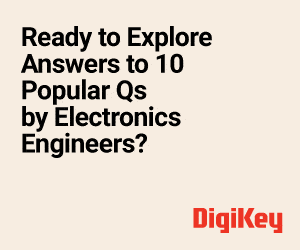
The proposed system was evaluated through a series of tests, during which it was used to generate patterns for approximately 5,000 textile samples composed of both natural and synthetic fabrics. The results demonstrated strong performance, with the system successfully producing accurate knitting instructions for the majority of the samples.
The model achieved an accuracy rate exceeding 97% in translating images into knitting instructions, significantly outperforming existing methods. It also demonstrated the ability to manage the complexity of multi-colored yarns and rare stitch types—areas where previous approaches had notable limitations. This advancement enables the possibility of fully automated textile production, offering reductions in both time and labor costs.
The newly developed model is expected to undergo further testing and refinement. In the future, it could be deployed in real-world manufacturing environments, potentially enabling large-scale, automated production of customized knitted garments. When integrated with knitting robotics, the system may allow designers to rapidly prototype or test new patterns without the need for manual generation of machine-readable instructions.
Future development plans include addressing dataset imbalances—particularly for infrequent stitch types—through advanced data augmentation techniques. Additional goals involve incorporating color recognition to enhance both structural and visual accuracy, enabling the system to handle variable input and output sizes, and extending the workflow to support the creation of complex 3D knitted garments. Broader applications in related domains such as weaving and embroidery are also under exploration.