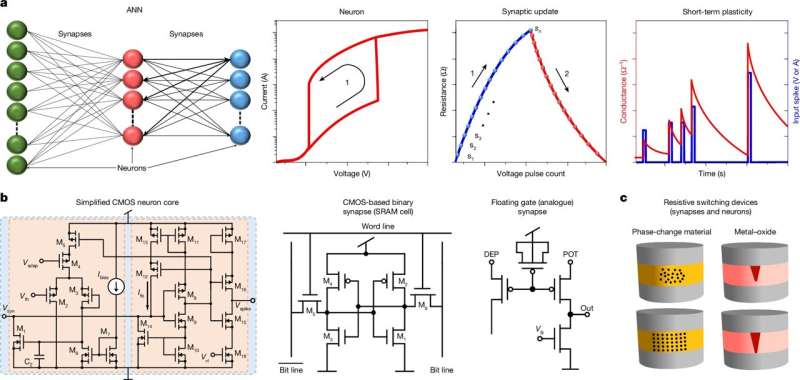
Check out our latest products
A new frontier in neuromorphic computing by demonstrating that a single silicon transistor can behave like a neuron and synapse, this advancement brings AI processors one step closer to mimicking the brain’s efficiency, paving the way for faster and more responsive computing.

A research team from the National University of Singapore (NUS) has made a breakthrough in neuromorphic computing by demonstrating that a single, standard silicon transistor can function like a biological neuron and synapse. Led by Associate Professor Mario Lanza from the Department of Materials Science and Engineering, this discovery could revolutionize artificial neural networks (ANNs) by making them more scalable and energy-efficient. Their study was published in Nature.
The human brain is vastly more energy-efficient than electronic processors, thanks to its 90 billion neurons and 100 trillion synapses that dynamically adjust their strength, a process known as synaptic plasticity. Scientists have long attempted to replicate this efficiency using ANNs, which power AI models like ChatGPT. However, software-based ANNs require vast computational resources and energy, limiting their practical applications.
– Advertisement –
Neuromorphic computing aims to overcome these limitations by designing chips that mimic the brain’s efficiency. This requires in-memory computing (IMC), where data storage and processing occur in the same place, and hardware that accurately replicates biological neurons and synapses.
A Major Step Forward in AI Hardware
Current neuromorphic systems rely on complex transistor arrays or experimental materials that lack large-scale manufacturing feasibility. The NUS team has now demonstrated that a single silicon transistor, when operated in a specific way, can replicate both neural firing and synaptic weight changes—the key mechanisms of biological neurons and synapses.
By fine-tuning the transistor’s resistance, they controlled two key physical phenomena: punch-through impact ionization and charge trapping. They also developed a two-transistor unit called “Neuro-Synaptic Random Access Memory” (NS-RAM), capable of switching between neuron-like and synapse-like behaviors.
Unlike other approaches requiring exotic materials, this method leverages commercial CMOS technology, the foundation of modern computer processors. This ensures scalability, reliability, and compatibility with existing semiconductor manufacturing.
The NS-RAM demonstrated low power consumption, stable performance over multiple cycles, and consistent behavior, making it a promising solution for energy-efficient AI hardware. This advancement paves the way for compact, power-efficient processors that could enable faster and more responsive computing in real-world applications.